INTRODUCTION
Plant diseases pose a continuing threat to food security, plant health, and ecosystem health globally (Jeger et al. 2021; Ristaino et al. 2021; Rizzo et al. 2021). Historically, plant disease epidemics and outbreaks have been associated with increased interconnectedness of the landscape, climate change, human movement, and increased intensification of crop production practices such as irrigation. Plant pathogens are responsible for an estimated 20-40% of crop losses, although net effects are hard to quantify due to a lack of evidence related to precise distribution and impact (Savary et al. 2019, 2022). Even so, even less is known about the prevalence of pathogens of minor crops, of those of regional importance only, of non-food crops or concerning the provision of ecosystem services from native wild plant populations and communities (Gougherty and Davies 2021, Jeger 2021). It is reasonable to assume that epidemics or major disease outbreaks in major crops are at least somewhat well documented, but what is often missing is comprehensive information on the extent and frequency of new outbreaks across all plant pathosystems. Here we test a potential approach to fill that knowledge gap by exploring approaches to retrieve information related to new plant outbreaks and epidemics from literature and database searches.
This review has two main objectives. The first objective is to provide a synoptic view of plant disease outbreaks across all hosts as reported in peer-reviewed scientific articles published in 2021. The second objective is to summarise information from a comprehensive database on new reports of pathogens spreading or emerging in new geographical regions – or on new host species – in 2021. Our working hypothesis was that there would be little overlap in the results obtained from meeting the two objectives of our study, mainly because it is reasonable to assume that new reports of a pathogen species will usually precede epidemics or major outbreaks of that species that might subsequently occur. Nevertheless, by accessing information from two complementary approaches, we anticipate that this will identify any limitations in identifying emerging and re-emerging plant diseases by automated literature reviews and/or database searches.
Objective 1. A literature search was made to identify articles in which the objectives of the study and the underlying methodology, particularly the use of surveys and other surveillance methods, are well-described. As we describe below, the key importance of such studies lies in providing information that goes beyond that obtained in reports of the presence or absence of a given disease. We accept that restricting the review to publications from just a single year, 2021, may be considered a limitation, but the periods referred to often span multiple years. However, difficulties in obtaining up-to-date information on epidemics/outbreaks may affect decision-making in disease management (Thompson et al., 2018; Severns & Mundt, 2021). A wider problem is how to correct for inevitable time lags between data collection, analysis, and reporting (Bastos et al., 2019).
The breadth of our ambition makes it impractical to use expert-based assessment methods (Savary et al. 2019), important though these may be for estimating global crop losses of major crops. We do not limit the review to major food crops, or indeed any plant host category, or to pathogens that experts consider to be important. Instead, we use methods accounting for disease outbreaks reported in the scientific literature irrespective of geographical regions, hosts, or causal organisms. In the latter case, comprehensive reviews have been published for most pathogen taxonomic groupings, for example, viruses and viroids (Jones, 2021), fungi and oomycetes (Fones et al., 2020), bacteria and mollicutes (Agrios 2005), and nematodes (Bernard et al., 2017). The methods we use are based on principles of a systematic review, and so recognise the need to: (i) avoid as much bias as possible in the choice of pathogens or hosts; (ii) identify pathogen species in the search and not specify them a priori because of their perceived importance; and (iii) avoid giving undue emphasis to pathogens or plants which are used as model systems or for presenting novel scientific interest.
We note that, in searching the scientific literature, it is important to recognise the different terminologies used. For example, ‘harmful organisms’ (a term used in plant health and pest risk analysis, Devorshak, 2012) is a generic term, including pests and weeds. Pests could also be used to refer to both invertebrate organisms that cause damage due to, for example, herbivory, and pathogens causing disease. The term parasite can be used to describe a wide range of symbiotic associations, from mutualistic to pathogenic (Jiang et al., 2020). The term pathogen is used to describe a parasite that causes disease, although there can be some fluidity depending on the biotic and abiotic environment (Méthot and Alizon, 2014). Disease itself often requires both a pathogen and a vector for it to manifest. A vector may itself be a pest in the absence of the pathogen. For this reason, it is important that the results of automated searches are scrutinised to confirm their relevance to the search strings used. Plant pathology is also still influenced – and arguably over-influenced – by the ‘one pathogen-one host’ paradigm (Lamichhane and Venturi, 2015). However, disease complexes, mixed infections, the co-occurrence of closely related species (a major problem with plant viruses), sub-species, and other intraspecific designations of variety, biovar, pathovar and forma specialis, are all known to occur. These factors complicate disease assessments, risk assessments and indeed systematic reviews. For this reason, we have been careful to reflect these complexities in our methodology and interpretation of results.
Objective 2. The second objective of the review reflects the distinction between outbreaks of long-established and well-characterised pathogens, and new reports of pathogens, sometimes poorly or newly characterised, spreading or emerging in new geographical regions or hosts (Bebber et al. 2013, 2014). Such reports often arise from the requirements of national and regional plant health regulations for official surveys to determine the presence or absence of a named harmful organism, usually at the species level. For new reports across all hosts, pathogens, and geographical regions, the use of a comprehensive database is essential to assess patterns that emerge from records made in any given year, in our case 2021.
The comprehensive database we use here is the CABI Distribution Database (DDB). This database holds over 1.2 million geographic records of organisms and their locations, with a focus on species which are important to agriculture and the environment. Over 55,000 species are represented, across key areas such as crop pests, crop and animal diseases, invasive species, and natural enemies. Most of the data has been sourced from peer reviewed published literature and other authoritative third parties such as plant protection organisations. Whenever available, information is stored on the origin, extent of distribution and the invasive status. The DDB was used for this review as it has the most comprehensive global coverage of plant pests and diseases relevant to agriculture, and since it is continuously updated to reflect changing species distributions.
DISCUSSION
Although yield losses in major crops are increasingly well documented (Savary et al. 2019, 2022), the underlying disease dynamics driving losses are much less well studied. Diseases in minor crops, non-food crops and natural plant systems are particularly poorly characterised. Here we used two contrasting approaches to analyse articles published in 2021 on plant disease epidemics across the world. The first (Objective 1) was a systematic review of the 2021 literature reporting surveys of plant disease occurrences. The second (Objective 2) retrieved new plant pathogen presence records added to the CABI Distribution Database (DDB) in 2021.
The approaches are complementary, with both helping to build a holistic picture of the range of plant health threats that are impacting, or have the potential to impact, food security, as well as plant and environmental health. In turn, information such as this can inform appropriate surveillance measures or management strategies to either prevent pathogens from entering a novel location or limit the likelihood or impact of recurring outbreaks.
Geographically, both approaches revealed the highest numbers of outbreaks and new records in North America, Asia, and Europe, with lower numbers in Africa and South America. There were also similarities in the pathogen taxa identified, with fungi and viruses dominating. Interestingly, however, the two approaches led to a pair of relatively distinct lists of specific pathogen species. Exceptions to this were
Candidatus Liberibacter asiaticus,
Citrus tristeza virus,
Colletotrichum siamense,
Fusarium oxysporum f. sp.
cubense Tropical race 4,
Globodera rostochiensis and
Tomato brown rugose fruit virus. These pathogens were retrieved from both approaches and of them
Ca. Liberibacter asiaticus,
Citrus tristeza virus and
Fusarium oxysporum f. sp.
cubense were reported in at least 5 publications in the 2021 literature (Objective 1,
Table 1).
Which Limitations Might Affect Our Methodology?
In Objective 1, we concentrated on published literature reporting the results of surveys. This task would have been made easier if journals specified general guidance for good practice in planning, designing, carrying out, analysing, and writing papers reporting the results of this type of survey. Such guidance would include reporting the rationale for surveys, the purposes they serve, the questions being asked, and whether they are hypothesis-driven or exploratory: often this vital information was lacking from the literature returned by our searches. Ideally, this guidance would also encourage at least a partial move away from single pathogen surveys, given the increasing recognition of disease complexes and syndromes and the recent awareness of mixed or co-infections and cryptic species (Lamichhane and Venturi, 2015). The increased splitting into various sub-specific forms and other genomic characterisations arising from surveys presents additional major data analysis challenges and difficulties in biological interpretation.
In Objective 2, we initially extracted records automatically from the DDB, but manual intervention was required to identify confirmed first reports of pathogens in new geographic locations or on new hosts. This is unsurprising since confirmed new reports are not the only focus of the DDB. Records required manual review for various reasons, predominantly due to taxonomic revisions and/or differences in the date of addition to the DDB versus the date of the survey itself. On other occasions, the literature cited by records found in the automated stage discusses surveys carried out with the purpose of determining pathogen prevalence, disease severity or pathogen impacts in locations where a given pathogen is already well known to occur. This was not our focus here, and so such reports required manual removal.
Certainly, pathogen distributions present a constantly evolving picture, and databases as used in Objective 2 can only possibly ever provide a snapshot in time. For example, Tomato brown rugose fruit virus was first reported as present in 2021 in Estonia, but has subsequently been reported as absent, having been eradicated (EPPO, 2022). It is also notable that the first reports from Objective 2 contain little to no information about the scale of crop losses caused by the pathogens identified. There is a paucity of quantitative data on the impacts of pathogens on their host plants. However, the publication of first reports is a vital first step in monitoring the subsequent impacts that occur.
Which Factors Might Bias Our Results?
Both approaches adopted here were systematic, explicitly designed to attempt to avoid bias toward certain crops or pathosystems. Results, therefore, ought to reflect the balance of current outbreaks and new findings. However, there are nevertheless unavoidable biases that remain. Both the literature we searched in Objective 1, and most of the literature and records contributing to the DDB in Objective 2, were in English, introducing a “Tower of Babel” bias into our results (Grégorie et al., 1995). This has been acknowledged to affect the results of past studies using similar sources of data (Bebber et al., 2019). Certain pathogens are topical for scientific reasons since they constitute model systems for experiments, for example A. thaliana and P. syringae (e.g., Karasov et al. 2018). The pathogens that appear in the literature are also driven, in part, by the need to attract research funding, or indeed the availability of funding, and do not necessarily reflect the impact of a disease in economic, social, or environmental importance.
Additionally, our results will have been affected by the way in which regulatory surveys for quarantine pests in plant health are principally targeted at substantiating claims for pest freedom. Such surveys could even be argued to provide perverse incentives which, in fact, reward under-sampling of those pests. Though risk and statistically based survey approaches are increasingly being adopted (EFSA 2020, Mastin et al., 2020), detection surveys are often used to establish presence/absence rather than providing representative surveys of disease dynamics. Lack of resources for representative and widespread monitoring of disease has in many cases led to significant outbreaks being undiscovered for decades. For example, Xylella fastidiosa was first reported in France in 2015, but independent studies have estimated it was introduced in the 1980s (Soubeyrand et al., 2018; Dupas et al., 2022). In the UK, ash dieback disease was first reported in 2012 but may have been present and spread from ash stock planted as early as 1991 (Wylder et al., 2018).
Our results likely underestimate the importance of pathogens in the “Global South”, reflecting greater surveillance and research effort in richer, developed countries (Jones et al., 2008). Certain pathogens have significant regulatory implications following first discovery, for example, Tomato brown rugose fruit virus, and others receive increased attention because of the impacts they might cause. Conversely, pathogens sometimes do not receive very much attention because they are perceived as being unimportant. This can have unintended consequences. As an example, there is a paucity of records on Xylella fastidiosa on deciduous tree species in North America. This likely reflects underreporting of the disease in these areas due to perceived low impact but has led to considerable current uncertainty in Europe as to what the impact could be if X. fastidiosa spreads in forest tree species present in the EU (EFSA 2019).
The structure of the geographic regions in the DDB also introduced some possibility for bias; for example, Ca. Liberibacter asiaticus was reported for the first time in Bahia, a state in Brazil. This only showed up in our results as a new outbreak because Brazil is one of the countries for which sub-national geographical resolution is retained in the DDB. A similar issue underpinned our identification of apple rubbery wood viruses as a pathogen presenting the signature of emergence/spread: the pathogen is certainly spreading, but all records in the DDB in 2021 relate to different locations in (mainland) China.
Other issues that impact on reporting of disease include a potential over-representation of those diseases that have strong symptom expression and existing tools for diagnosis, as well as time lags between when samples are collected in the field and when laboratory diagnosis occurs. Moreover, our approach does not capture data from the any number of regulatory surveys that are not published nor reported via the DDB, which might particularly affect pathogens which are not notifiable.
Implications for Disease Management
Studies concerned with aspects of disease management accounted for many of the articles recovered under Objective 1, very often linked to disease surveillance in regulated zones (Lazaro et al. 2021) and detection/ discrimination of new pathogens or novel strains using molecular diagnostics. Examples include Sri Lankan cassava mosaic virus emerging in Southeast Asia (Charoenvilaisiri et al. 2021), Xylella subspp in the Balearic Islands (Olmo et al. 2021), citrus tristeza virus strains (Park et al. 2021, Llanes-Alvarez et al. 2021), Erwinia spp in the USA (Singh et al. 2021), antibiotic resistance in Pseudomonas syringae pv actinidiae (Psa) (Lee et al., 2021), fungicide resistance in Zymoseptoria tritici (Garnault et al., 2021) (see detailed summaries in Electronic Supporting Information, S5). Many studies were concerned with the field performance and deployment of resistant varieties: such as for pokkah boeng disease in sugarcane (Shan et al. 2021, Solic-Palacios et al. 2021), wheat yellow rust (Perronne et al. 2021, Esmail 2021). Fewer field studies were made on the use of biological control agents in disease management: such as for root knot nematode in vegetable crops (Sabri et al. 2021), Psa in kiwi fruit (Ares et al. 2021) (Electronic Supporting Information, S5).
From a broader perspective, disease management is increasingly seen as a multifunctional issue involving the securing of food supplies while safeguarding ecosystem health and reducing dependency on natural resources (He et al. 2021), including the extended use of within-crop diversification (Wang et al. 2021b) as shown for Zymoseptoria tritici in intraspecific mixed stands of wheat varieties (Orelana-Torrejon et al., 2021; Electronic Supporting Information, S5). Integrated crop management in both low- and high-input cropping systems is seen as an instrument for maintaining yields while reducing reliance on synthetic inputs such as pesticides and fertilizers (Richard et al. 2021). The use of even intermittent conventional insecticides to control Diaphorina citri, the vector of Ca. Liberibacter asiaticus (Clas) may affect the activity of natural enemies (Shrestha et al. 2021; Electronic Supporting Information, S5). Studies on Ca. Liberibacter asiaticus (Clas) in feral understorey citrus in forested areas have suggested that shading may have the potential to reduce disease transmission in commercial citrus production (Vincent et al. 2021: Electronic Supporting Information, S5). Disease management may differ between optimal and sub-optimal areas: such as for Fusarium oxysporum f. sp. cubense (Foc) in banana cultivation (Teixeira et al., 2021), maize lethal necrosis (MLN) and maize seed productions systems in different agro-ecological zones (Eunice et al., 2021) (Electronic Supporting Information, S5).
Where containment is practised as a disease management objective, the importance of involving a broad spectrum of participants – farmers, extension workers, practitioners, and plant health regulators – is now well recognised (Ladisa et al. 2021). Such involvement is necessary to improve cooperation and coordination amongst participants (Sherman et al. 2019) and should form part of any risk-based approach to disease management (Hyatt-Twynam et al. 2017). These concerns were mirrored in studies on Clas in California (Garcia-Figuera et al., 2021), cassava mosaic virus disease in Zambia (Szyniszewska et al. 2021) (Electronic Supporting Information, S5). Other studies on disease management of Clas have given detailed accounts of control techniques such as precision trunk inoculation but have not considered the implications for all participants (Li and Nangong, 2021: Electronic Supporting Information, S5).
The complexities of disease management where there are multiple pathogens present in a mixed or rotational cropping system are illustrated for maize-soybean systems (Hampf et al. 2021, Meier et al. 2021), rice-wheat/maize systems (Shan et al., 2021b, Sekiya et al. 2022), and grapevine-cover crop systems (Léon et al., 2021) (Electronic Supporting Information, S6). However, in general, this important topic is arguably under-represented in the literature, given the real world context in which many growers are placed.
On detecting the first report of a pathogen, some publications identified under Objective 2 emphasised the requirement to monitor and control vector populations that may facilitate the spread of pathogens. This was a particular concern for disease management of Cucurbit chlorotic yellows virus in Texas, USA, where whiteflies in the Bemisia tabaci species complex are abundant (Hernandez et al. 2021). Publications also speculated on the possible sources of infection by novel pathogens, with many suggesting they originated from the importation of commercial seed (Sabra et al. 2021). It was also highlighted that for Tomato brown rugose fruit virus, the movement of unregulated seed and propagation by keen gardeners may be another pathway of introduction and a further threat to commercial crop production (Hamborg and Blystad 2022, cited by EPPO 2021). All these aspects need to be taken into consideration to develop robust management strategies to prevent introductions, to quickly identify them when they do occur and to limit their subsequent spread and potential crop losses.
Some first reports in Objective 2 were identified from samples of asymptomatic plants, often the result of official surveys carried out as part of a routine monitoring program. Such programs are more likely to be established for pathogens known to be high risk, such as Tomato brown rugose fruit virus in Europe and Candidatus Liberibacter asiaticus in Citrus growing regions of Brazil (EPPO 2021). They can also be particularly useful in detecting pathogens that might otherwise have spread undetected due to their symptoms being consistent with those of other, perhaps more common, pathogens (Chynoweth et al. 2021). Another category of first reports represented the outcome of pre-export examinations of both commercial and non-commercial seed (EPPO, 2021 for Tomato brown rugose fruit virus; Skelton et al. 2019, cited by EPPO 2021 for Potato spindle tuber viroid). These studies highlight the critical role border inspections of imports and exports play in preventing spread of novel pathogens.
Predicting Future Spread of Disease
Often linked to disease management, disease prediction assumes that being able to predict future disease risk and occurrence can lead to improved disease management or choice of disease control options. Predictions may be relevant at different temporal and spatial scales, and with different levels of statistical sophistication, from individual fields or production units, based on weather data (Kim et al. 2020, Shah et al. 2019) and/or phenological data (Hjelkrem et al. 2021) to the landscape level (Yuen and Mila, 2015). Prediction may relate to individual pathogen species or, more generally, to taxa with varying levels of vulnerability based on phylogenetic relationships (Robles-Fernández & Lira-Noriega 2017). Neural network models and standard daily meteorological data gave the best prediction of the population dynamics of D. citri, the vector of CLas, at the field scale in Pakistan (Bibi et al. 2021; Electronic Supporting Information, S5). Typical field-based prediction models linked to weather data and crop phenology/morphology were developed for B. tabaci, the vector of cassava mosaic viruses, in Uganda (Katono et al. 2021; Electronic Supporting Information, S5). A weather-based model was developed to predict when a threshold would be reached for use of a systemic fungicide to control Z. tritici (Beyer et al. 2022; Electronic Supporting Information, S5).
Risk-based prediction at the regional level has become more common in recent years and is most relevant to the topic of this paper. Risk-based surveillance methods have been developed for early detection of invasive plant pathogens. The perhaps counter-intuitive result is that basing surveillance only on areas predicted to be high risk is not always the optimal strategy (Mastin et al. 2020). A model was developed to predict the probability of pine wood nematode occurrence in Hubei province, China, based on vegetation condition, anthropogenic activity and topographic features obtained from remote sensing data and geographic information systems (Zhang et al. 2021; Electronic Supporting Information, S5). Distinct subpopulations of the nematode occur in China; based on genomic variation, it was predicted that the nematode survives better at lower temperatures than previously thought (Ding et al. 2021; Electronic Supporting Information, S5). Ecological niche modelling was used to predict the likely occurrence in regions of Mexico of Trioza erytreae, a vector of Ca. Liberibacter africanus (Claf) as well as Clas (Espinoza-Zaragoza et al., 2021; Electronic Supporting Information, S5). Species distribution models have also predicted that in future years climatic suitability may be less favourable for Philaenus spumarius, the main vector of X. fastidosa in Europe (Godefroide et al. 2022; Electronic Supporting Information, S5).
Climate Change, Yield & Ecosystem Services
Disease prediction may prove of limited value unless the impact of climate change on crops, pathogens, vectors, and the broader biotic environment are taken into consideration (Lops 2022, Sreenivas 2022). This broader view, however, often provides general opinion rather than specific detail. In some cases, detailed experimental information on the host-pathogen interaction in relation to temperature gives some indication of likely responses to a global climate (e.g., Amari et al. 2021). Historical data over extended periods have been used to predict trends in future years and countries for specific diseases or groups of pathogens of crops and wild plants (Bebber et al. 2019, Burdon & Zhan 2020, Jeger 2021, Chen et al. 2022, Tang et al. 2017). In a comprehensive review, Chaloner et al. (2021) tracked the likely global trends in crop production, yields, and disease pressure. These authors predicted that yield and infection risk will increase at high latitudes over the 21st century, but that in the tropics there will be limited productivity gain and a reduction in infection risk. The balance between yield gains and infection risk in relation to climate change and variability makes the consequences for food security uncertain. A process-based simulation model was used to predict the incidence and severity of rice blast disease under different time scenarios and climate change scenarios (Wang et al. 2021b; Electronic Supporting Information, S5). Little change was predicted by 2030, with a slight decline by 2070. A key factor in modulating trends in disease was the level of cultivar resistance. However, no predictions were made on the impacts on yields.
The avoidance of crop loss due to disease is a major motivation for making improvements in disease management and prediction and for securing global food supplies (Savary et al. 2012, Savary et al. 2019, Savary and Willoquet, 2020), especially for pathogens of the major staple food crops such as wheat and rice (Ficke et al. 2018, Savary et al. 2022). Household surveys in Kenya have shown very high losses in maize due to maize lethal necrosis (MLN), approaching 80% in the western highland tropical and moist transitional zones (de Groote et al. 2021; Electronic Supporting Information, S5). Fewer quantitative data are available for pathogens of minor or speciality food crops, including pome fruit and grapevine (Trad and Taoueb 2021, Frem et al. 2021; Electronic Supporting Information, S5) although, because of higher per unit horticultural value, estimates of economic loss have been made. Losses of forest trees in nurseries or young plantations have often been expressed in terms of % mortality (Thu et al. 2021; Electronic Supporting Information, S6).
Analogous to yield in wild plant populations and communities is the provision of ecosystem services (Paseka et al. 2020, Windsor et al. 2021, Cavender-Bares et al. 2022, Nowak-Olejnik et al. 2022) recognising that there may also be ecosystem disservices, i.e., negative effects (Guo et al. 2022). Losses in the provision of ecosystem services are then analogous to crop losses. However, this aspect has rarely been reported, and most studies have concentrated on the role of wild plants as reservoirs or alternate hosts of pathogens infecting crop or cultivated plants (Mugerwa et al., 2021, Doolotkeldieva et al. 2021, Pak et al., 2021, Regassa et al., 2021, Chen et al., 2021; Electronic Supporting Information, S5).
Outlook
Clearly, more investment is needed in plant health monitoring, not just to accurately characterise presence or absence at a country level, but also to monitor spatial distribution and temporal changes for improved epidemiological intelligence (Ristaino et al., 2021). This is sorely needed for rapid responses to new and emerging diseases, particularly in low- and middle-income countries where the impacts can be particularly severe (Carvajal-Yepes et al., 2019). Better sources of data on disease presence and particularly absence, would allow predictive mathematical models to be properly parameterised, which remains challenging in practice (Cunniffe et al., 2015). It would also allow novel outbreaks of re-emerging diseases which can no longer be controlled in traditional ways (Bhattacharya et al., 2017) to be targeted. New pathogen detection technologies, for example with the application of remote sensing (Zarco-Tejada et al., 2018) are being developed, and could potentially feed into more cost effective and widespread monitoring. There are also opportunities to include diffuse data sources into the information stream concerning the spread of pathogens, including, for example, citizen science (Brown et al., 2020), landowner reports (Defra, 2020) and results of horizon scanning activities by regulators (EFSA, 2022).
This study has confirmed the value of two complementary approaches to identifying current plant disease outbreaks and potential new risks through publications in the peer-reviewed scientific literature (Objective 1), and new reports in an extensive database (Objective 2). These two approaches give different perspectives on current and future plant disease threats. It is noteworthy that only three pathogens, Ca. Liberibacter asiaticus, citrus tristeza virus and Fusarium oxysporum f. sp. cubense, were identified in both approaches, reflecting their continuing impact in areas they have long been established and their spread to new areas where previously absent. Six of the 14 pathogens reported extensively in 2021 in the scientific literature are vectored by insects. The role of vectors in disease transmission, epidemiology and spread needs as much attention as the pathogen. A good example of this concern is the African citrus psyllid Trioza erytreae, first recognised as having spread to Europe in the last decade (Cocuzza et al. 2017), and which now poses the risk of allowing huanglongbing to establish. Although the pathogens identified are well-known and characterised, they (or their vectors) continue to spread. The question remains which of the pathogens identified as new records in databases will in future years cause major disease outbreaks and be reported extensively in the scientific literature.
Very few pathogens spread intercontinentally through natural dispersal, mostly rust fungi such as sugarcane rust and cereal rusts (Jeger 1999, Brown and Hovmøller 2002). In many cases the role of international trade in plants and plant products can seed subsequent establishment, spread and impact of pathogens (Brasier, et al. 2008, Jeger et al. 2021). A traded plant may carry a pathogen to a new location, or a naïve introduced plant may acquire a new pathogen in the new location. Similarly, a vector may move with the traded plant, or an invasive pathogen may acquire a new vector. Numerous examples of the introductions of exotic and emerging pathogens can be found in the listings of pathogens identified in Objective 2 of this review. Finally, problems remain of delays in reporting, publishing, and communicating plant disease outbreaks and new records for plant health regulation, disease management and farm strategic planning. We suggest that the approach taken in this review provides a mechanism for integrating information and a synoptic view on current plant disease outbreaks and potential future risks and might usefully be adopted regularly in the future.
Figure 1.
Methodology for the survey of published articles on disease outbreaks and epidemics. The workflow and number of results after each stage of filtering for the review of the scientific literature published in 2021. Numbers represent the number of identified articles at each stage: the final review after all exclusions were applied summarises n = 186 articles.
Figure 1.
Methodology for the survey of published articles on disease outbreaks and epidemics. The workflow and number of results after each stage of filtering for the review of the scientific literature published in 2021. Numbers represent the number of identified articles at each stage: the final review after all exclusions were applied summarises n = 186 articles.
Figure 2.
Review of records in the CABI Distribution Database (DDB). The workflow and number of results from the exploration of the DDB for new species occurrence records added to the database in 2021. Numbers represent the number of identified records which consist of a concept and the related geographic area. Totals are also shown for the number of unique geographic areas and concepts at each stage of the process. A total of 28 pathogens had four or more new occurrence records in 2021 according to DDB. It was followed by manual review of all pathogens with more than 4 new records (n = 28), to identify confirmed first reports in a new location (n=15). “Concept”a = A generic name for an entity in the distribution database for which location and presence/absence data are stored. Most commonly an organism recorded at species level, but in some cases recording occurs at higher or lower taxonomic levels depending on data available. “Geographic area”b = One of 507 defined regions covering the globe of national or sub-national (e.g., state, province) administrative areas and other regions, islands and sea areas of biogeographic importance.
Figure 2.
Review of records in the CABI Distribution Database (DDB). The workflow and number of results from the exploration of the DDB for new species occurrence records added to the database in 2021. Numbers represent the number of identified records which consist of a concept and the related geographic area. Totals are also shown for the number of unique geographic areas and concepts at each stage of the process. A total of 28 pathogens had four or more new occurrence records in 2021 according to DDB. It was followed by manual review of all pathogens with more than 4 new records (n = 28), to identify confirmed first reports in a new location (n=15). “Concept”a = A generic name for an entity in the distribution database for which location and presence/absence data are stored. Most commonly an organism recorded at species level, but in some cases recording occurs at higher or lower taxonomic levels depending on data available. “Geographic area”b = One of 507 defined regions covering the globe of national or sub-national (e.g., state, province) administrative areas and other regions, islands and sea areas of biogeographic importance.
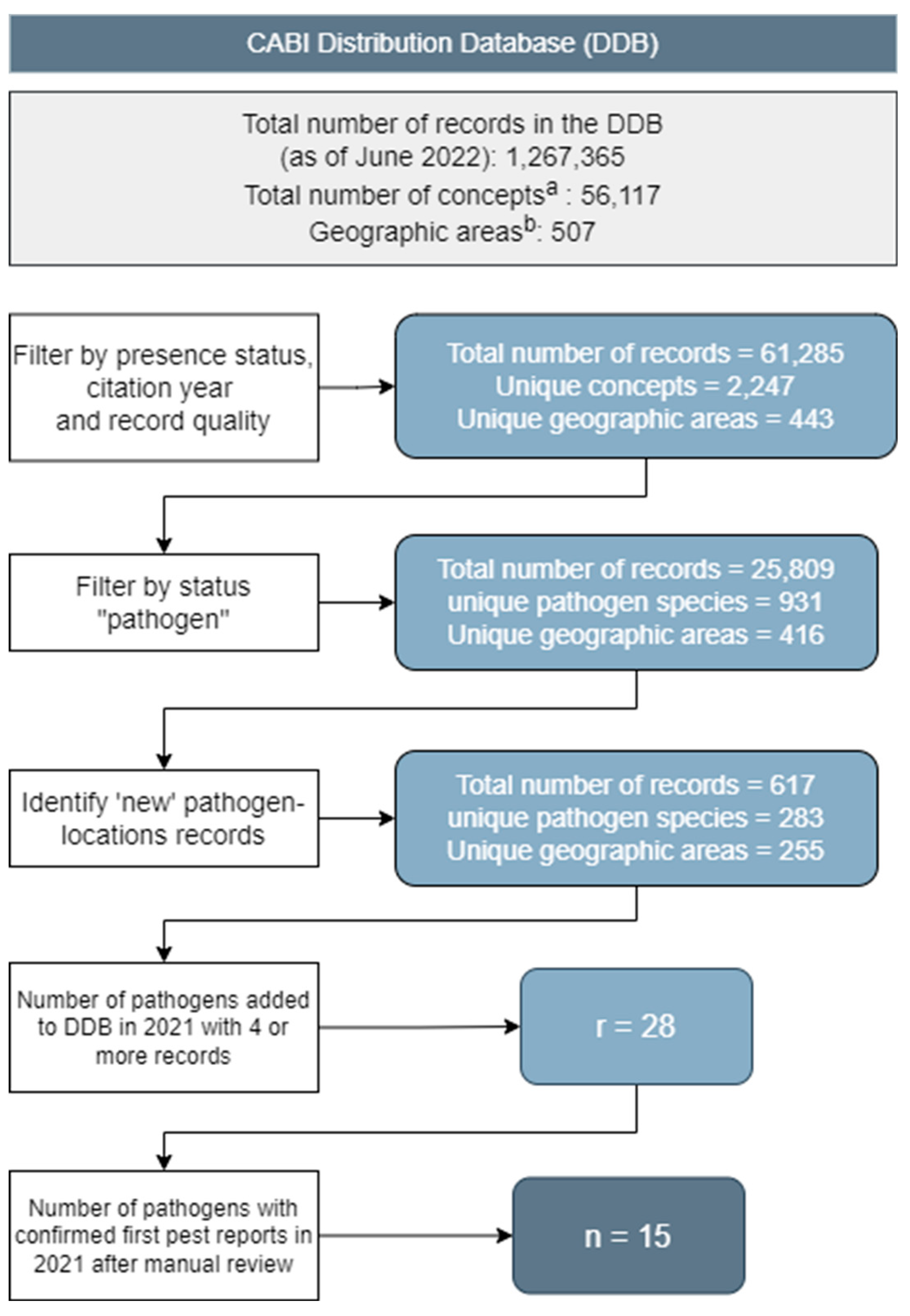
Figure 3.
Categorisation of core articles on single named species identified in Stage 1 of the review of published articles on disease outbreaks and epidemics. A – Number of host plants per continent where retrieved pathogen species were observed. B – Number of pathogen species per group of pathogens and host plants on which they were observed. C – Number of pathogen species per continent and study descriptor. D – Number of reported species grouped by study descriptors and pathogen group.
Figure 3.
Categorisation of core articles on single named species identified in Stage 1 of the review of published articles on disease outbreaks and epidemics. A – Number of host plants per continent where retrieved pathogen species were observed. B – Number of pathogen species per group of pathogens and host plants on which they were observed. C – Number of pathogen species per continent and study descriptor. D – Number of reported species grouped by study descriptors and pathogen group.
Figure 4.
Map showing number of pathogen-location pair records retrieved from core articles on single named species and multiple pathogens identified in Stage 1 of the review of published articles on disease outbreaks and epidemics. The number of records by continent and species type of pathogen. Two studies had global remit, therefore n=2 was added to each continent’s totals. A map showing country by country data is available in Electronic Supporting Information, S3 (Figure S3-1).
Figure 4.
Map showing number of pathogen-location pair records retrieved from core articles on single named species and multiple pathogens identified in Stage 1 of the review of published articles on disease outbreaks and epidemics. The number of records by continent and species type of pathogen. Two studies had global remit, therefore n=2 was added to each continent’s totals. A map showing country by country data is available in Electronic Supporting Information, S3 (Figure S3-1).
Figure 5.
Symptoms caused by the diseases identified in the review of published articles on disease outbreaks and epidemics. A) Fusarium oxysporum f. sp. cubense (Panama disease of banana); symptoms on a banana plant (Public Domain - Released by Scot Nelson/via flickr - CC0); B) Meloidogyne enterolobii (Pacara earpod tree root-knot nematode); rootknot symptoms on an ornamental Petunia species (©Jeffrey W. Lotz/Florida Department of Agriculture & Consumer Services/Bugwood.org - CC BY 3.0 US); C) Cassava mosaic disease (African cassava mosaic); symptoms on cassava leaf. (©Phil Taylor, CABI); D) Citrus tristeza virus (©Tim Gottwald); E) Erwinia amylovora (fireblight) on pear (©Phil Taylor, CABI); F) Candidatus Liberibacter asiaticus (Asian greening); HLB symptoms. April 2016 (©Nian Wang (Citrus Research and Education Center)); G) Pseudomonas syringae pv. syringae (bacterial canker) symptoms on peach (Prunus persica), bark peeled to show discoloration beneath (©University of Georgia Plant Pathology/University of Georgia/Bugwood.org - CC BY 3.0 US); H) Fusarium on wheat (©Phil Taylor, CABI); I) Magnaporthe oryzae (rice blast) in Myanmar (©Phil Taylor, CABI); J) Puccinia striiformis f. sp. tritici; symptoms of yellow rust on triticale leaf (©Rasbak/via Wikimedia Commons - CC BY-SA 4.0); K) Zymoseptoria tritici, septoria on wheat (©Phil Taylor, CABI); L) Symptoms of maize lethal necrosis disease (©Joseph Mulema, CABI); M) Bursaphelenchus xylophilus (pine wilt nematode); pine wilt symptoms, one year after death of tree (©USDA Forest Service/Region 2- Rocky Mountain Region/USDA Forest Service/Bugwood.org - CC BY 3.0 US); N) Symptoms of Xyella fastidiosa on olive in Apulia, Italy, 2015 (©Dr Stephen Parnell).
Figure 5.
Symptoms caused by the diseases identified in the review of published articles on disease outbreaks and epidemics. A) Fusarium oxysporum f. sp. cubense (Panama disease of banana); symptoms on a banana plant (Public Domain - Released by Scot Nelson/via flickr - CC0); B) Meloidogyne enterolobii (Pacara earpod tree root-knot nematode); rootknot symptoms on an ornamental Petunia species (©Jeffrey W. Lotz/Florida Department of Agriculture & Consumer Services/Bugwood.org - CC BY 3.0 US); C) Cassava mosaic disease (African cassava mosaic); symptoms on cassava leaf. (©Phil Taylor, CABI); D) Citrus tristeza virus (©Tim Gottwald); E) Erwinia amylovora (fireblight) on pear (©Phil Taylor, CABI); F) Candidatus Liberibacter asiaticus (Asian greening); HLB symptoms. April 2016 (©Nian Wang (Citrus Research and Education Center)); G) Pseudomonas syringae pv. syringae (bacterial canker) symptoms on peach (Prunus persica), bark peeled to show discoloration beneath (©University of Georgia Plant Pathology/University of Georgia/Bugwood.org - CC BY 3.0 US); H) Fusarium on wheat (©Phil Taylor, CABI); I) Magnaporthe oryzae (rice blast) in Myanmar (©Phil Taylor, CABI); J) Puccinia striiformis f. sp. tritici; symptoms of yellow rust on triticale leaf (©Rasbak/via Wikimedia Commons - CC BY-SA 4.0); K) Zymoseptoria tritici, septoria on wheat (©Phil Taylor, CABI); L) Symptoms of maize lethal necrosis disease (©Joseph Mulema, CABI); M) Bursaphelenchus xylophilus (pine wilt nematode); pine wilt symptoms, one year after death of tree (©USDA Forest Service/Region 2- Rocky Mountain Region/USDA Forest Service/Bugwood.org - CC BY 3.0 US); N) Symptoms of Xyella fastidiosa on olive in Apulia, Italy, 2015 (©Dr Stephen Parnell).
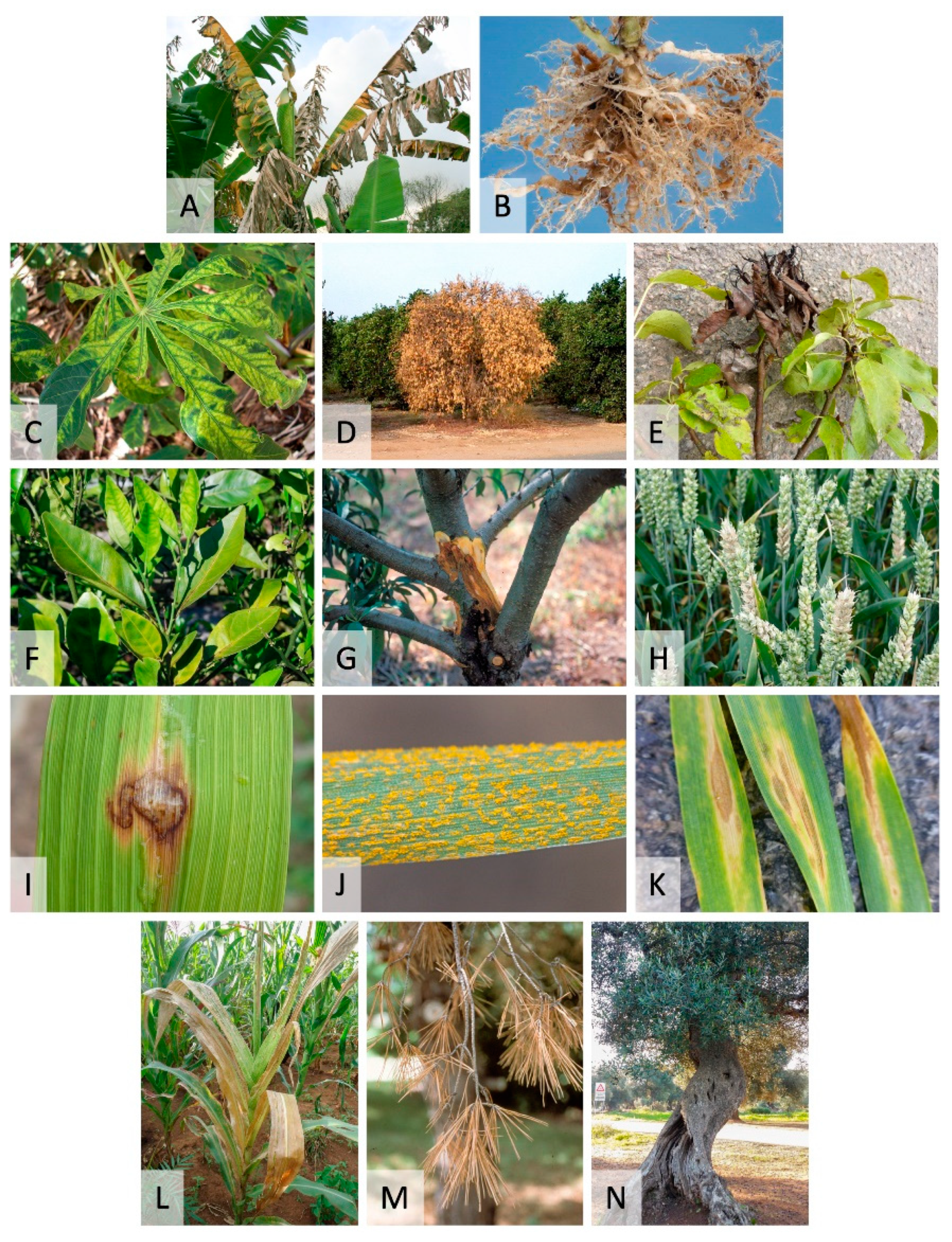
Figure 6.
Unique pathogen-host pairs in new pathogen records reported in 2021 in the CABI Distribution Database. Host species were identified in each location where new pathogen records were added to DDB in 2021 and were reported in four or more geolocations defined in DDB. One or more host species could be identified in each geolocation. Host species were classified in groups, and locations by continent.
Figure 6.
Unique pathogen-host pairs in new pathogen records reported in 2021 in the CABI Distribution Database. Host species were identified in each location where new pathogen records were added to DDB in 2021 and were reported in four or more geolocations defined in DDB. One or more host species could be identified in each geolocation. Host species were classified in groups, and locations by continent.
Figure 7.
Map showing locations of new pathogen records reported in 2021 in the CABI Distribution Database following manual review. Counts are summarised by continent, with a breakdown of pathogen type by kingdom. A map showing country by country data is available in Electronic Supporting Information, S3 (Figure S3-2).
Figure 7.
Map showing locations of new pathogen records reported in 2021 in the CABI Distribution Database following manual review. Counts are summarised by continent, with a breakdown of pathogen type by kingdom. A map showing country by country data is available in Electronic Supporting Information, S3 (Figure S3-2).
Figure 8.
Map showing combined locations of new pathogen records reported in 2021 from both the literature review and from the CABI Distribution Database (CDD) (following manual review). Counts at CDD geolocation level are summarised by continent, with a breakdown of pathogen type by kingdom. A map showing country by country data is available in Electronic Supporting Information, S3 (Figure S3-3).
Figure 8.
Map showing combined locations of new pathogen records reported in 2021 from both the literature review and from the CABI Distribution Database (CDD) (following manual review). Counts at CDD geolocation level are summarised by continent, with a breakdown of pathogen type by kingdom. A map showing country by country data is available in Electronic Supporting Information, S3 (Figure S3-3).
Table 1.
Combined results following Stage 1 and 2 from the review of published articles on disease outbreaks and epidemics. Summaries of the results for each of these categories are given in the main text; the range of survey dates for the named species/complexes is also indicated.
Table 1.
Combined results following Stage 1 and 2 from the review of published articles on disease outbreaks and epidemics. Summaries of the results for each of these categories are given in the main text; the range of survey dates for the named species/complexes is also indicated.
NAMED SPECIES OR SPECIES COMPLEX |
|
|
|
Number of articles |
Year/s of survey |
|
Candidatus Liberibacter asiaticus and vectors |
10 |
2009-2019 |
|
Cassava mosaic viruses |
10 |
2013-2020 |
|
Xylella fastidiosa and vectors |
10 |
2011-2020 |
|
Erwinia amylovora |
7 |
2015-2020 |
|
Puccinia striiformis f. sp. tritici
|
7 |
1985-2020 |
|
Zymoseptoria tritici |
7 |
1999-2020 |
|
Bursaphelenchus xylophilus |
7 |
2000-2020 |
|
Meloidogyne spp. complexes |
6 |
2013-2019 |
|
Magnaporthe oryzae |
6 |
2003-2019 |
|
Citrus tristeza virus |
6 |
2012-2019 |
|
Fusarium spp. complexes |
6 |
2015-2019 |
|
Fusarium oxysporum f. sp. cubense
|
5 |
2016-2017 |
|
Pseudomonas syringae pvs |
5 |
1982-2019 |
|
Maize lethal necrosis co-infecting viruses |
5 |
2013-2019 |
MULTIPLE PATHOGENS/CROPPING PRODUCTION PRACTICE |
|
|
|
Number of articles |
|
|
Aquaculture |
5 |
|
|
Crop-associated weeds |
5 |
|
|
Cropping systems |
5 |
|
|
Forest tree pathogens |
5 |
|
|
Wild plants |
5 |
|
Table 2.
New pathogen records reported in 2021 in the CABI Distribution Database. Of the 28 pathogens which met the threshold for manual review – i.e., had at least four new records – the 15 shown above were confirmed as constituting new reports of a named pathogen in a new location. The "Percentage of records from 2021” column was calculated by taking “New records in 2021 after manual review” as a percentage of “Pre 2021 records” + “New records in 2021 after manual review”. This allowed us to designate four pathogens as exhibiting a signature of actively emerging and/or spreading: pathogens for which the percentage of records from 2021 was >25% of the total and for which there were at least 2 records in 2021 after manual review. These pathogens are discussed in the main text.
Table 2.
New pathogen records reported in 2021 in the CABI Distribution Database. Of the 28 pathogens which met the threshold for manual review – i.e., had at least four new records – the 15 shown above were confirmed as constituting new reports of a named pathogen in a new location. The "Percentage of records from 2021” column was calculated by taking “New records in 2021 after manual review” as a percentage of “Pre 2021 records” + “New records in 2021 after manual review”. This allowed us to designate four pathogens as exhibiting a signature of actively emerging and/or spreading: pathogens for which the percentage of records from 2021 was >25% of the total and for which there were at least 2 records in 2021 after manual review. These pathogens are discussed in the main text.
PATHOGEN |
NUMBER OF DDB RECORDS |
|
|
Pre 2021 records |
New 2021 records |
2021 records after manual review |
Percentage of new records from 2021 |
Emerging and/or spreading? |
Tomato brown rugose fruit virus |
18 |
16 |
15 |
45.45% |
✓ |
Cucurbit chlorotic yellows virus |
24 |
12 |
11 |
31.43% |
✓ |
apple rubbery wood viruses |
27 |
8 |
8 |
22.86% |
|
Aphelenchoides besseyi |
130 |
8 |
4 |
2.99% |
|
Apple hammerhead viroid |
1 |
5 |
4 |
80.00% |
✓ |
Colletotrichum siamense |
0 |
4 |
4 |
100.00% |
✓ |
Potato spindle tuber viroid |
56 |
5 |
3 |
5.08% |
|
Erysiphe corylacearum |
16 |
4 |
3 |
15.79% |
|
Erwinia rhapontici |
10 |
8 |
1 |
9.09% |
|
Citrus tristeza virus |
160 |
7 |
1 |
0.62% |
|
Globodera rostochiensis |
99 |
7 |
1 |
1.00% |
|
Biscogniauxia mediterranea |
3 |
5 |
1 |
25.00% |
|
Fusarium oxysporum f. sp. cubense Tropical race 4 |
31 |
5 |
1 |
3.13% |
|
Candidatus Liberibacter asiaticus |
105 |
4 |
1 |
0.94% |
|
Nothophoma quercina |
2 |
4 |
1 |
33.33% |
|