1. Introduction
Today, the world consumes 14 billion cubic meters of concrete annually, equating to approximately 4.4 tons per individual [
1]. Despite its popularity, concrete has some inherent difficulties that limit its use under harsh weather conditions and in modern architectural designs. A major drawback of concrete is its relatively low tensile strength. Reinforced concrete (RC) structures are traditionally designed without considering this property, constituting approximately 6 to 12% of the normal concrete’ compressive strength (CS) [
2,
3,
4]. Using discrete, randomly distributed, and discontinuous steel fibers in concrete reinforces the mix to mitigate problems [
5,
6,
7]. This type of composite material is known as steel fiber-reinforced concrete (SFRC). Consequently, RC members can be enhanced in crack resistance by using this easy-to-manufacture and highly effective technology [
8].
Concrete stands out as the foremost building material employed extensively across the global construction industry, thanks to its unparalleled attributes of durability, strength, and sustainability [
9,
10,
11]. Within this context, Steel Fiber Reinforced Concrete (SFRC), fortified with short discrete fibers as mass reinforcement, emerges as a highly efficient cement-based composite capable of mitigating, to a considerable extent, the inherent brittleness of plain concrete [
12]. The incorporation of fibers into concrete represents a pivotal enhancement, notably augmenting its strength, toughness, crack resistance, and tension performance. This transformative effect occurs because the haphazardly distributed fibers adeptly curtail the unstable propagation of cracks, operating both at the micro and macro levels [
13]. Remarkably, SFRC exhibits a phenomenon known as strain-softening behavior, persisting even after the emergence of macro-cracks—a testament to the significant crack control offered by these fibers [
14]. The introduction of steel fiber reinforcement into concrete goes a step further, markedly elevating its ductility and toughness. This outcome is primarily attributed to the introduction of supplementary fracture mechanisms and the energy expended in overcoming the interlocking and adhesion forces between the fibers and the cementitious matrix [
15]. Assessing the enhancement of the ultimate properties necessitates mechanical tests and can only be determined upon reaching the conclusion of the loading phase. Recent developments have underscored the utility of acoustic emission as a means to evaluate the performance of SFRC beams [
16]. Additionally, it's worth noting that the formation of macro-cracks can potentially lead to the corrosion of steel reinforcement. Therefore, the reduction in crack width, achieved through the presence of fibers, assumes paramount importance in enhancing the durability of Reinforced Concrete (RC) structural elements [
17]. Furthermore, compelling evidence suggests that the inclusion of steel fibers may reduce the need for conventional steel shear reinforcement. This conclusion is drawn from a comprehensive array of tests and analyses conducted by the authors and other esteemed researchers in the field [
18,
19,
20].
The SFRC was patented by Bernard in 1874 for strengthening concrete in tension, using steel splinters to achieve this purpose [
21]. In the ensuing years, this exploration has led to many studies being conducted on its microstructure [
22,
23], flowability and SFRC’s behavior under tension [
24,
25], durability [
26,
27,
28], strength under extreme and cyclic loadings [
28,
29,
30]. In recent years, several new types of fibers have been proposed as reinforcements for the SFRC, along with the use of magnetic fields to align the steel fibers during casting [
30,
31], and microscale numerical analyses have been published to illustrate the fundamental failure mechanism of SFRC under varying external loads [
32,
33]. According to these studies, SFRC has substantially different strength and elasticity properties than traditional concrete. For the post-cracking response, the SFRC is positively influenced by the high tensile resistance and elasticity that results in a crack-bridging mechanism. Hence, this composite material has excellent behavior under tension and shear loadings. A large extent of mechanical property variability is, however, a result of material heterogeneity. A steel fiber reinforcement can prevent macrocrack propagation in concrete, but the resulting SFRC may be less flowable than its conventional equivalent. The quality reduction is likely caused by the interference between aggregates and fibers [
33].
A design-oriented formulation for the key mechanical properties of SFRCs is necessary for the calculation of their key performance characteristics to facilitate their successful implementation in practical applications. Further, the existing models, however, tend to be either insufficiently accurate or lack physical relevance. In the literature, there have been several studies [
34,
35,
36] proposing linear empirical formulas for predicting SFRC mechanical properties. There is a general consensus that fiber dosage and water-binder ratio play the greatest role in determining SFRC behavior. A composite material mixing theory indicates that SFRC properties strongly correlate with matrix and steel fiber elastic properties. This theoretical background has been used as a foundation for the development of various empirical models [
37]. In spite of the fact that some of the currently available empirical models are capable of accurately predicting the results of a small number of tests [
7,
38], the development and implementation of comprehensive and robust models are still in the early stages of development.
Machine learning (ML) and ML interpretability algorithms find extensive application in various aspects of structural engineering. These applications span a wide spectrum of areas within structural engineering, encompassing: structural analysis and design, structural health monitoring and damage detection, fire resistance of structures, resistance of structural members under various loads, andthe characterization of mechanical properties and mix design of concrete [
39]. As an illustration of the capabilities of these techniques, Zheng et al. [
40] successfully employed a YOLO-v5 model to detect surface cracks on wind turbines. Similarly, Cardellicchio et al. [
41] leveraged ML to facilitate the recognition and interpretation of defects for the purpose of risk management in heritage bridge preservation.
The use of machine learning (ML) to model the SFRC's mechanical properties has become increasingly popular over the last few years. In this context, the back-propagation Artificial Neural Network (ANN) approach has been utilized to calculate the SFRC's CS by Açikgenç et al. [
38]. Their study used the aggregate size at maximum, fiber dosage, length, and size, as well as fiber length and size, as input variables. In a similar manner, Awolusi et al. [
42] used an ANN method to predict the flowability, CS, and splitting strength of SFRC. In addition, Karahan [
43] used multiple nonlinear regression techniques in conjunction with ANN to predict the long-term strength of SFRC containing varying levels of fly ash. While these closed-source databases may be able to provide accurate estimates of SFRC mechanical properties, their limitations remain a concern. There have been criticisms of these models for their deficiencies, which include a lack of a physical mechanism illustration and a lack of an application tool. Recently, Pakzad et al. [
7] have employed various data-driven machine-learning algorithms to predict the CS of SFRC. Sensitivity and parametric analyses were performed to demonstrate the capabilities of ML algorithms in their study.
In this study, we present solutions to the above-mentioned by focusing on the CS of SFRC-containing mono-fibrous (crimped, hooked, and mil-cut) systems. The research has been completed in several phases to achieve the goal. First, a comprehensive database of 420 records collected from 43 published studies was compiled and refined to establish a representative sample population. The second component of this research involves constructing and evaluating a numerical model that uses a state-of-the-art ML technique (XG Boost). A third aspect of the study involved narrowly focused experiments designed to verify the developed model using 30 SFRC mixes. Fourth, the model parameters were ranked according to their importance, and partial dependence plots were constructed to visualize their relationships. An intuitive graphical user interface was developed to enhance the model's applicability.
ML has seen increased application in modeling SFRC properties. However, many current ML models for this purpose are "black boxes" that lack transparency and physical relevance, and also present implementation challenges. Moreover, globally, there is significant consumption of concrete, which, despite its popularity, has inherent limitations, including its relatively low tensile strength. The necessity to improve the transparency, relevance, and implement-ability of ML models for SFRC properties, combined with the global demand for more durable and versatile concrete solutions, drives this research. Existing models for predicting and optimizing SFRC properties using ML lack transparency, do not have a clear physical basis, and are challenging to implement in practical scenarios. This study introduces an innovative approach that utilizes the extreme gradient (XG) Boosting algorithm for predicting and optimizing the CS of SFRC. The approach is grounded in a comprehensive database, which is a compilation from 43 publications, and is validated through extensive experimental studies. The ML model developed in this research not only promises superior predictive capabilities against independent experimental data but also introduces a user-friendly interface, paving the way for more accessible and efficient predictions and optimizations in the realm of SFRC. This study represents a transformative effort within the construction industry landscape. Although ML techniques have gained prominence in predicting material properties, a significant challenge endures—the lack of transparency and practical relevance in existing models. To address this challenge and enhance real-world applicability, this research adopts a meticulous approach, leveraging the XG Boosting algorithm. Furthermore, the investigation gains clarity through the application of Partial Dependence Plots (PDPs), which reveal intricate connections between input parameters and the CS of SFRC. Of utmost importance, the current study uncovers optimal SFRC formulations, offering practical insights into the most effective combinations of fibers for real-world applications.
6. Conclusions, implications, and future research
This study involved the compilation and refinement of an extensive database, comprising 420 entries sourced from 43 scholarly publications. We conducted experimental analyses on 20 different SFRC mixtures to assess the predictive accuracy of the constructed model. Furthermore, we employed PDPs to elucidate the relationships between the model's input variables and its outcomes. The significance of these input variables within the model was also explored. To enhance the model's usability, we developed a user-friendly graphical interface. It is worth noting that the research specifically focused on three distinct fiber types: crimped, hooked, and mil-cut. Therefore, the findings may not be directly applicable to SFRCs with alternative fiber types or mixtures. Additionally, while the model consistently performed well with experimental data, its effectiveness may vary under different conditions or when using different raw materials. Regarding the research findings:
1.The analysis, including Pearson correlations, Gini indices, and SHAP analyses, highlighted that the most significant factors influencing the CS of SFRC were the cement and HRWR contents, as well as the fiber tensile strength-water-binder ratio. Notably, increasing the proportion of water and coarse aggregates is likely to reduce the compressive strength of the concrete.
2. We utilized the Pareto frontier multi-criterion method to develop an optimized version of the standard XG Boost model. Based on training and testing datasets, the optimized model demonstrated satisfactory predictive performance, achieving scores of 0.97 and 0.88, respectively.
3. The developed ML model consistently exhibited superior predictive capability when tested against independent experimental data conducted by the authors, with average and COV values of the tested-predicted results at 0.99 and 6%, respectively.
4. Through the application of PDPs, we determined that the optimal water and HRWR contents for achieving maximum CS are in the range of 100–150 kg/m³ and 10–20 kg/m³, respectively. Similarly, for coarse aggregates, ideal contents fall in the ranges of 900–1100 kg/m³. Additionally, the most effective fibrous combination exhibited a tensile strength of 1000 MPa, a diameter length of 40–50 mm, and a dosage of about 1.0%.
The adoption of ML techniques, particularly the XG Boosting methodology, offers the construction and civil engineering sectors an enhanced predictive toolset for determining the CS of SFRC. This research not only elucidates optimal SFRC formulations, pinpointing effective fiber combinations but also facilitates the development of concrete with superior strength and durability. In future investigations, it would be valuable to compare the predictive competence of the current numerical model against existing empirical and analytical frameworks. Additionally, the inclusion of data on ultra-high-performance concrete could enhance the model's universality. Addressing the size effect might benefit from the incorporation of a conversion factor as an input variable for various types of test samples, effectively contributing to handling this aspect of the study. While our present research offers a robust database, there is a compelling case for expanding this repository by incorporating newer studies and a wider array of SFRC mix variations, ensuring it remains at the forefront of technological progress. Despite the current study's reliance on the XG Boosting technique, exploring alternative ML schemes (e.g., neural networks or stacked ensemble alogrithms) may reveal novel perspectives and enhance predictive accuracy. Beyond immediate CS predictions, there is a growing need to investigate the enduring resilience of SFRC in diverse scenarios. In an era emphasizing ecological responsibility, future research should critically assess the environmental implications of various SFRC formulations, including aspects such as lifecycle assessments, carbon emissions, and potential for recycling.
Figure 1.
XG Boost methodology.
Figure 1.
XG Boost methodology.
Figure 2.
Boxplots of the model variables.
Figure 2.
Boxplots of the model variables.
Figure 3.
A graphic depiction of a model's variables.
Figure 3.
A graphic depiction of a model's variables.
Figure 4.
(a) Pearson’s encoded matrix, and (b) linear correlations between features and label.
Figure 4.
(a) Pearson’s encoded matrix, and (b) linear correlations between features and label.
Figure 5.
Pareto frontier-based model’s multi-objective optimization.
Figure 5.
Pareto frontier-based model’s multi-objective optimization.
Figure 6.
Predicted vs. target results for the: (a) training, and (b) testing data.
Figure 6.
Predicted vs. target results for the: (a) training, and (b) testing data.
Figure 7.
SEM analysis and particle size curves for the used OPC.
Figure 7.
SEM analysis and particle size curves for the used OPC.
Figure 8.
Particle size curves for various aggregates.
Figure 8.
Particle size curves for various aggregates.
Figure 9.
The uniaxial compression test: (a) representatives’ diagram and (b) detailed setup.
Figure 9.
The uniaxial compression test: (a) representatives’ diagram and (b) detailed setup.
Figure 10.
Predicted vs. target results for experimental verification data.
Figure 10.
Predicted vs. target results for experimental verification data.
Figure 11.
Variable significance by: (a SHAP value, and (b) Gini index methods.
Figure 11.
Variable significance by: (a SHAP value, and (b) Gini index methods.
Figure 12.
Compressive strength of SFRC partial dependence plots.
Figure 12.
Compressive strength of SFRC partial dependence plots.
Figure 13.
A GUI for predicting the compressive strength of SFRC using the XG Boost model.
Figure 13.
A GUI for predicting the compressive strength of SFRC using the XG Boost model.
Table 1.
Coding system used for the dummy variables.
Table 1.
Coding system used for the dummy variables.
Fiber type |
Hooked |
Mil-cut |
Crimped |
|
1 |
0 |
0 |
|
0 |
1 |
0 |
Table 2.
Coding system used for other variables.
Table 2.
Coding system used for other variables.
variable |
Unit |
Description |
variable |
Unit |
Description |
|
kg/m3
|
Water content |
|
kg/m3
|
HRWR content |
|
Cement content |
|
MPa |
Tensile strength of the fiber |
|
–– |
water-binder ratio |
|
mm |
Diameter of the fiber |
|
kg/m3
|
Fine aggregate content |
|
%, vol. |
Dosage of the fiber |
|
Coarse aggregate content |
|
mm |
Length of the fiber |
|
mm |
Maximum aggregate size |
|
MPa |
28d CS of the SFRC |
Table 3.
Summary of the collected CS database of SFRC.
Table 3.
Summary of the collected CS database of SFRC.
Ref. |
Data-sets |
|
|
|
|
|
|
|
|
|
|
|
|
|
|
[53] |
17 |
1 |
0 |
180–192.5 |
280–400 |
0.5|0.6|0.7 |
713.8–762.6 |
1027.2–1097.4 |
31.5 |
3.4–6.6 |
–– |
0.19–0.64 |
0.55–0.9 |
30–60 |
29.7–46.7 |
[54] |
12 |
1 |
0 |
162–177 |
354–450 |
0.4|0.5 |
893–920 |
858–887 |
19 |
2.3–6.5 |
1050 |
0–1 |
0.75 |
60 |
31.5–53.3 |
[55] |
4 |
1 |
0 |
181.2 |
453 |
0.4 |
624 |
1242 |
22 |
1 |
1100 |
0–0.8 |
0.75 |
60 |
21.7–25.3 |
[56] |
5 |
1 |
0 |
230.2 |
338 |
0.7 |
1049.2 |
760 |
10 |
0.7 |
–– |
0–1.25 |
0.75 |
60 |
25.6–28.1 |
[57] |
40 |
1 |
0 |
175 |
350 |
0.5 |
952.2 |
1321.2–1398.8 |
20 |
4.5 |
1100–1250 |
0–1.5 |
0–1.5 |
50–60 |
17.9–40.8 |
[58] |
14 |
1 |
0 |
178–179 |
325–396 |
0.4|0.6 |
842–891 |
913–965 |
19 |
0.9–1.4 |
1050–2000 |
0–1 |
0.71–0.75 |
60 |
47.2–61.1 |
[59] |
2 |
1 |
0 |
175.6 |
351 |
0.5 |
914.8 |
994.7 |
22 |
2.7 |
–– |
0.26–0.45 |
1 |
50 |
33.3–33.4 |
[60] |
5 |
1 |
0 |
205–398 |
311–458 |
0.6|0.7 |
408–665 |
1156–1407 |
20 |
3.1–7.3 |
1700 |
0–1 |
0.4 |
25 |
31.2–88.0 |
[61] |
2 |
1 |
0 |
169.8–177.6 |
283–296 |
0.6 |
705–838 |
968–1071 |
10 |
2.8–3.3 |
–– |
1–1.5 |
0.62 |
30 |
23.3–30.0 |
[62] |
7 |
1 |
0 |
220 |
440 |
0.5 |
1193–1225 |
356–366 |
10 |
3–4 |
–– |
0–1.5 |
0.75 |
25–31 |
35.1–43.8 |
[63] |
8 |
1 |
0 |
191.2–191.6 |
324 |
0.6 |
1052.8–1064.2 |
750.0–758.3 |
22 |
3.2 |
1200 |
0.19–0.76 |
0.5–0.75 |
30–60 |
34.0–48.0 |
[64] |
2 |
1 |
0 |
185 |
410 |
0.5 |
915 |
866 |
20 |
2–4 |
1100 |
0.5–1.0 |
1 |
50 |
50.0–52.0 |
[65] |
10 |
0|1 |
0 |
150–168 |
341–429 |
0.3|0.4|0.5 |
800 |
975 |
14 |
2.8–11 |
–– |
0.51 |
1 |
54–60 |
40.9–67.6 |
[66] |
18 |
1 |
0 |
123–167 |
325–439 |
0.6 |
741–885 |
846–998 |
14 |
0.5–11.4 |
–– |
0.48–0.75 |
1 |
54–60 |
38.2–69.6 |
[36] |
6 |
1 |
0 |
195 |
342 |
0.3|0.5|0.6 |
701 |
1143 |
20 |
10.3 |
–– |
0–1.5 |
0.75 |
30 |
24.6–30.6 |
[67] |
23 |
1 |
0 |
164–185 |
308.3–529.1 |
0.3|0.6 |
639.3–763.9 |
928.6–1232.7 |
20 |
0–5.8 |
600–1000 |
0-2 |
0.55–0.75 |
35 |
26.7–65.4 |
[68] |
32 |
1 |
0 |
172–195 |
336–521 |
0.6 |
652–750 |
1080–1145 |
10–40 |
0–5.2 |
1100 |
0-1 |
0.75 |
30–60 |
25.8–67.4 |
[69] |
21 |
0|1 |
0 |
166 |
286 |
0.4 |
739 |
1170–1259 |
20 |
0–0.6 |
600 |
0–2 |
0.75 |
30–40 |
28.0–33.0 |
[35] |
5 |
0 |
0 |
165 |
367 |
0.3|0.4|0.5 |
702–765 |
1053–1146 |
16 |
2.2 |
380 |
0–2 |
0.5 |
30 |
26.8–31.0 |
[70] |
12 |
1 |
0 |
195–200 |
415–651 |
0.4|0.7 |
527–610 |
1022–1182 |
20 |
0 |
1000 |
0–1.5 |
0.75 |
32 |
30.9–58.5 |
[71] |
6 |
1 |
0 |
185 |
268–524 |
0.4|0.5|0.6 |
488–725 |
1056–1185 |
31.5 |
0 |
1000 |
0–0.63 |
1 |
50 |
16.0–34.4 |
[72] |
22 |
0|1 |
0|1 |
172–196 |
321–475 |
0.4|0.5 |
622.8–886.0 |
960–1171 |
20 |
0–4.5 |
–– |
0–2 |
0.75–0.94 |
32.3–62 |
26.6–43.6 |
[73] |
6 |
1 |
0 |
156–165 |
312–381 |
0.6 |
817–1200 |
700–1214 |
20 |
2.2–5.3 |
1000 |
0.38–0.77 |
1 |
50 |
63.9–67.5 |
[74] |
13 |
0|1 |
0 |
264 |
480 |
0.4 |
716.5–769.1 |
895.1–989.5 |
20 |
0 |
380–500 |
0–2 |
0.9–1.2 |
30.2–32.3 |
27.7–34.3 |
[75] |
4 |
1 |
0 |
175 |
461 |
0.4 |
512 |
1252 |
10 |
0 |
600 |
0–1.5 |
1.0 |
35 |
28.5–31.4 |
[76] |
2 |
1 |
0 |
160 |
400 |
0.4|0.6 |
750 |
1140 |
20 |
8 |
1700 |
0–0.5 |
0.5 |
30 |
42.7–45.1 |
[77] |
2 |
1 |
0 |
230 |
535 |
0.5 |
556 |
1079 |
16 |
0 |
1345 |
0–1.0 |
0.6 |
30 |
36.5–41.1 |
[37] |
2 |
0 |
0 |
165 |
300–471 |
0.4 |
690–760 |
1038–1140 |
20 |
1.8–2.8 |
380 |
1 |
0.5 |
30 |
23.8–39.0 |
[78] |
18 |
0 |
1 |
195–228 |
361–475 |
0.4 |
630–875 |
715–1180 |
20 |
0 |
380 |
0–2 |
0.94 |
32.2 |
28.1–34.1 |
[79] |
2 |
1 |
0 |
166 |
415 |
0.4 |
838.6 |
1024.9 |
20 |
0 |
1345 |
0–1 |
0.55 |
35 |
40.8–42.6 |
[80] |
5 |
0 |
1 |
161 |
460 |
0.4 |
1150 |
1048.8 |
20 |
18.4 |
808.6 |
0–1 |
0.8 |
32 |
30.0–40.6 |
[81] |
4 |
0 |
1 |
215.5 |
480 |
0.4 |
825 |
880 |
25 |
8 |
–– |
0–0.51 |
0.5 |
38 |
40.6–49.3 |
[82] |
16 |
0|1 |
0|1 |
160–200 |
258–513 |
0.4|0.5|0.6 |
540–868 |
1012–1283 |
20 |
0 |
500–1325 |
0–0.7 |
0.55–1.15 |
32–50 |
27.0–47.0 |
[83] |
5 |
1 |
0 |
172 |
400 |
0.4 |
730 |
1046–1100 |
15 |
0 |
–– |
0 |
0.8 |
32 |
27.7–37.0 |
[84] |
10 |
0 |
0 |
161.0–167.7 |
453.3–460.0 |
0.4 |
699.2 |
1594.2 |
20 |
18.1–18.4 |
808.6 |
0–1.6 |
0.8 |
32–40 |
43.5–56.0 |
[85] |
28 |
1 |
0 |
161.7 |
437 |
0.4 |
756 |
1210 |
20 |
1.3–6.1 |
–– |
0–1.5 |
0.7 |
35–50 |
31.8–50.3 |
[86] |
3 |
1 |
0 |
168.1 |
410 |
0.4 |
1073 |
645 |
16 |
4.1–5.7 |
1100 |
0–1 |
0.8 |
50 |
32.1–38.7 |
[87] |
3 |
1 |
0 |
167.7 |
390 |
0.4 |
1075 |
758 |
16 |
2.3–3.1 |
1100 |
0–1.45 |
0.8 |
50 |
36.9–40.5 |
[88] |
9 |
1 |
0 |
198–205 |
440–460 |
0.4|0.5 |
924–985 |
721–846 |
12 |
11.1–12.8 |
1225 |
0.76 |
0.62 |
40 |
52.4–70.0 |
[89] |
5 |
1 |
0 |
165 |
300 |
0.6 |
1128.8 |
806.3 |
16 |
2.5 |
1050–1100 |
0–0.5 |
0.55–0.75 |
35–60 |
17.6–29.2 |
[90] |
6 |
1 |
0 |
190 |
380 |
0.6 |
1082.0 |
742.0 |
12 |
0.0–2.4 |
2200 |
0–0.63 |
0.35 |
30 |
21.6–43.92 |
[91] |
3 |
1 |
0 |
169.1 |
412.4 |
0.4 |
927.8 |
890.7 |
12 |
0.0 |
1100 |
0–0.63 |
1 |
50 |
40.1–41.6 |
[92] |
3 |
1 |
0 |
188.0 |
400.0 |
0.5 |
610.0 |
1132.0 |
10 |
3.3 |
1270 |
0–1 |
0.62 |
30 |
36.4–40.5 |
Tot. |
422 |
0|1 |
0|1 |
123–398 |
258–651 |
0.3–0.7 |
408–1225 |
356.0–1594.2 |
10–40 |
0.0–18.4 |
380–2200 |
0–2 |
0.1–1.2 |
25–62 |
16–88 |
Table 4.
Data consistency conversion factors.
Table 4.
Data consistency conversion factors.
Specimen type |
Conversion factor |
Source |
Note |
Cubic |
0.80 |
ACI 318 [93] |
Below 60 MPa strength |
Cubic |
0.90 |
ACI 318 [93] |
Above 60 MPa strength |
Prismatic |
0.96 |
Wu et al. [94] |
–– |
Table 5.
Descriptive statistics of the processed datasets.
Table 5.
Descriptive statistics of the processed datasets.
Variable |
Mean |
StDev |
Minimum |
Q1 |
Median |
Q3 |
Maximum |
|
0.8012 |
0.3997 |
0.0000 |
1.0000 |
1.0000 |
1.0000 |
1.0000 |
|
0.0865 |
0.2814 |
0.0000 |
0.0000 |
0.0000 |
0.0000 |
1.0000 |
|
181.19 |
23.62 |
123.00 |
165.00 |
175.00 |
192.50 |
264.00 |
|
394.97 |
74.01 |
258.00 |
342.00 |
392.00 |
450.00 |
651.00 |
|
0.472 |
9.23 |
0.285 |
0.390 |
0.4800 |
0.550 |
0.690 |
|
804.05 |
152.58 |
488.00 |
713.00 |
756.00 |
911.00 |
1225.00 |
|
1067.6 |
210.6 |
356.0 |
945.0 |
1088.6 |
1206.3 |
1594.2 |
|
19.542 |
5.218 |
10.000 |
19.000 |
20.000 |
20.000 |
40.000 |
|
3.451 |
4.131 |
0.000 |
0.000 |
2.750 |
4.963 |
18.400 |
|
989.4 |
378.5 |
380.0 |
600.0 |
1050.0 |
1200.0 |
2200.0 |
|
0.7246 |
0.5377 |
0.0000 |
0.3840 |
0.5780 |
1.0000 |
2.0000 |
|
0.7553 |
0.1970 |
0.1000 |
0.7000 |
0.7500 |
0.9000 |
1.2150 |
|
44.015 |
12.178 |
25.000 |
32.300 |
40.000 |
60.000 |
62.000 |
|
39.677 |
11.034 |
16.000 |
31.800 |
37.418 |
45.358 |
70.000 |
Table 6.
XG Boost hyperparameters for the initial and fine-tuned models.
Table 6.
XG Boost hyperparameters for the initial and fine-tuned models.
Hyperparameter |
Role |
Range |
Default value (Model-0) |
Optimized value (Model-1) |
max_depth |
Tree Maximum Depth: Modifying this parameter to higher values results in a more intricate model, increasing the risk of overfitting. |
|
6 |
52 |
n_estimators |
This hyperparameter dictates the quantity of boosting iterations or trees incorporated within the ensemble. |
|
100 |
325 |
learning_rate |
step size shrinkage employed during updates is intended to mitigate overfitting |
0–1 |
0 |
0.2 |
colsample_bytree |
The subsample ratio of columns determines the proportion of features used when constructing each tree. |
0–1 |
1 |
0.1 |
subsample |
Subsample ratio of training instance (e.g., 0.5 indicates 50% of data used prior to growing trees). |
>0–1 |
1 |
0.5 |
reg_alpha |
L1 regularization (increasing its value makes the model more conservative). |
–– |
0 |
0.01 |
reg_lambda |
L2 regularization (increasing its value makes the model more conservative). |
–– |
1 |
10 |
gamma |
Regularization parameter for tree pruning that specifies the minimum loss reduction required to make a split. |
|
0 |
0.1 |
Table 7.
Performance metrics of the initial and fine-tuned models.
Table 7.
Performance metrics of the initial and fine-tuned models.
Performance indicator |
Model-0 |
Model-1 |
Training set |
Testing set |
Training set |
Testing set |
|
0.742 |
3.541 |
1.239 |
2.797 |
|
2.576 |
28.413 |
4.008 |
16.997 |
|
1.605 |
5.330 |
2.002 |
3.933 |
|
0.978 |
0.776 |
0.966 |
0.879 |
Table 8.
The physicochemical properties of the used OPC.
Table 8.
The physicochemical properties of the used OPC.
Oxide composition (%) |
L.O.I. (%) |
Specific gravity |
Fineness (m2/kg) |
SiO2
|
Al2O3
|
Fe2O3
|
CaO |
MgO |
Na2Oeq |
SO3
|
20.20 |
5.49 |
4.12 |
65.43 |
0.71 |
0.26 |
2.61 |
1.38 |
3.14 |
373 |
Table 9.
Properties of the used steel fibers.
Table 9.
Properties of the used steel fibers.
Fiber |
Length (mm) |
Diameter (mm) |
Aspect ratio |
Young’s Modulus (GPa) |
Tensile strength (MPa) |
shape |
ID |
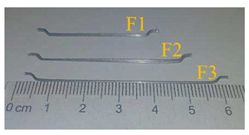 |
F1 |
40 |
0.62 |
65 |
210 |
1250 |
F2 |
50 |
0.62 |
80 |
F3 |
60 |
0.75 |
80 |
Table 10.
Test features and response.
Table 10.
Test features and response.
|
|
Model’s input variables |
|
|
No. |
ID |
|
|
|
|
|
|
|
|
|
|
|
|
|
|
|
1 |
H-F1-0.5 |
1 |
0 |
157.5 |
450 |
0.35 |
716 |
1053 |
10 |
0.68 |
1250 |
0.62 |
0.5 |
65 |
69.4 |
74.1 |
0.937 |
2 |
N-F1-0.5 |
1 |
0 |
157.5 |
350 |
0.45 |
798 |
1078 |
10 |
0.42 |
1250 |
0.62 |
0.5 |
65 |
60.7 |
56.3 |
1.078 |
3 |
H-F1-1.0 |
1 |
0 |
157.5 |
450 |
0.35 |
716 |
1053 |
10 |
0.68 |
1250 |
0.62 |
1.0 |
65 |
72.3 |
65.8 |
1.099 |
4 |
N-F1-1.0 |
1 |
0 |
157.5 |
350 |
0.45 |
798 |
1078 |
10 |
0.42 |
1250 |
0.62 |
1.0 |
65 |
64.1 |
61.8 |
1.037 |
5 |
H-F1-1.5 |
1 |
0 |
157.5 |
450 |
0.35 |
716 |
1053 |
10 |
0.68 |
1250 |
0.62 |
1.5 |
65 |
75.9 |
79.7 |
0.952 |
6 |
N-F1-1.5 |
1 |
0 |
157.5 |
350 |
0.45 |
798 |
1078 |
10 |
0.42 |
1250 |
0.62 |
1.5 |
65 |
62.3 |
59.1 |
1.054 |
7 |
H-F2-0.5 |
1 |
0 |
157.5 |
450 |
0.35 |
716 |
1053 |
10 |
0.68 |
1250 |
0.62 |
0.5 |
80 |
73.8 |
71.7 |
1.029 |
8 |
N-F2-0.5 |
1 |
0 |
157.5 |
350 |
0.45 |
798 |
1078 |
10 |
0.42 |
1250 |
0.62 |
0.5 |
80 |
55.8 |
60.4 |
0.924 |
9 |
H-F2-1.0 |
1 |
0 |
157.5 |
450 |
0.35 |
716 |
1053 |
10 |
0.68 |
1250 |
0.62 |
1.0 |
80 |
76.4 |
81.2 |
0.941 |
10 |
N-F2-1.0 |
1 |
0 |
157.5 |
350 |
0.45 |
798 |
1078 |
10 |
0.42 |
1250 |
0.62 |
1.0 |
80 |
62.3 |
61.1 |
1.020 |
11 |
H-F2-1.5 |
1 |
0 |
157.5 |
450 |
0.35 |
716 |
1053 |
10 |
0.68 |
1250 |
0.62 |
1.5 |
80 |
77.3 |
80.3 |
0.963 |
12 |
N-F2-1.5 |
1 |
0 |
157.5 |
350 |
0.45 |
798 |
1078 |
10 |
0.42 |
1250 |
0.62 |
1.5 |
80 |
64.2 |
69.6 |
0.922 |
13 |
H-F3-0.5 |
1 |
0 |
157.5 |
450 |
0.35 |
716 |
1053 |
10 |
0.68 |
1250 |
0.75 |
0.5 |
80 |
68.0 |
63.2 |
1.076 |
14 |
N-F3-0.5 |
1 |
0 |
157.5 |
350 |
0.45 |
798 |
1078 |
10 |
0.42 |
1250 |
0.75 |
0.5 |
80 |
58.9 |
60.7 |
0.970 |
15 |
H-F3-1.0 |
1 |
0 |
157.5 |
450 |
0.35 |
716 |
1053 |
10 |
0.68 |
1250 |
0.75 |
1.0 |
80 |
70.6 |
68.9 |
1.025 |
16 |
N-F3-1.0 |
1 |
0 |
157.5 |
350 |
0.45 |
798 |
1078 |
10 |
0.42 |
1250 |
0.75 |
1.0 |
80 |
59.7 |
61.8 |
0.966 |
17 |
H-F3-1.5 |
1 |
0 |
157.5 |
450 |
0.35 |
716 |
1053 |
10 |
0.68 |
1250 |
0.75 |
1.5 |
80 |
78.6 |
83.4 |
0.942 |
18 |
N-F3-1.5 |
1 |
0 |
157.5 |
350 |
0.45 |
798 |
1078 |
10 |
0.42 |
1250 |
0.75 |
1.5 |
80 |
66.2 |
68.6 |
0.965 |
19 |
H-CTRL |
1 |
0 |
157.5 |
450 |
0.35 |
716 |
1053 |
10 |
0.68 |
–– |
–– |
–– |
–– |
61.7 |
59.8 |
1.032 |
20 |
N-CTRL |
1 |
0 |
157.5 |
350 |
0.45 |
798 |
1078 |
10 |
0.42 |
–– |
–– |
–– |
–– |
52.4 |
57.2 |
0.916 |
|
|
|
|
|
|
|
|
|
|
|
|
|
|
|
|
|
0.992 |
|
|
|
|
|
|
|
|
|
|
|
|
|
|
|
|
|
0.058 |
|
|
|
|
|
|
|
|
|
|
|
|
|
|
|
|
CV |
0.059 |